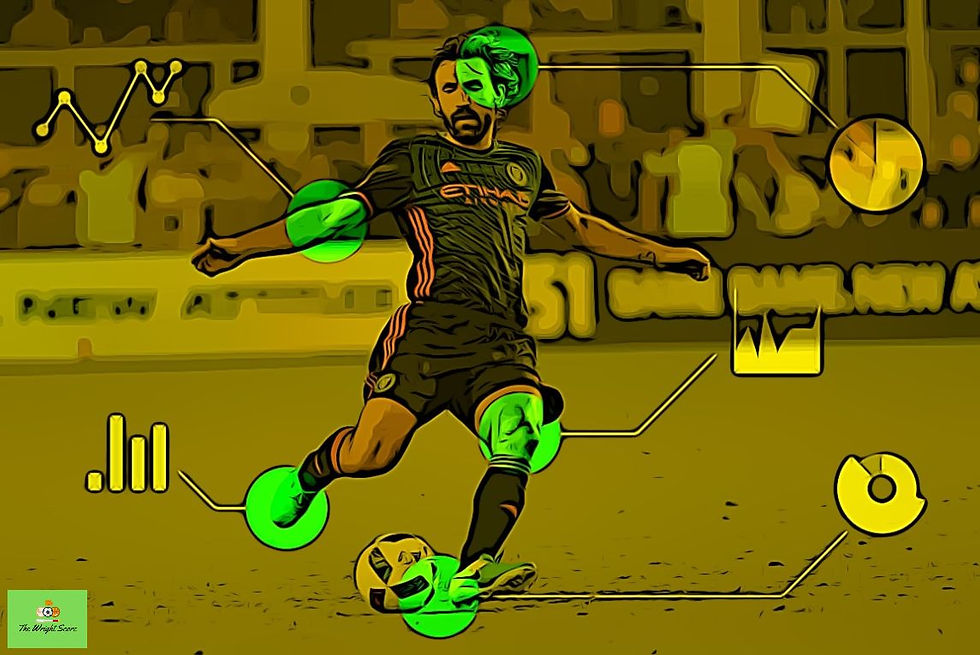
Data Analytics and statistics have taken over the industry of athletics. Nearly every sport has what we call advanced analytics to help determine a team's efficiency or how to evaluate player performance.
However, the one sport that is still looking for their breakthrough is soccer. The game of soccer is an extremely hard sport to analyze. The reason for this is because, on average, the game is very low-scoring. One-fourth of the competitions ends in a tie, while 8% go without a single goal being scored. The other reason is that the game is so fluid. There are no stop and starts, unlike baseball and football
Below is a breakdown of the key statistics in baseball, basketball and football. After, I will show my research on soccer's key data and show the significance they have or lack thereof by running a regression in R-studio and examine the R-squared and p-value of each statistic used during a typical soccer game.
Baseball Statistics
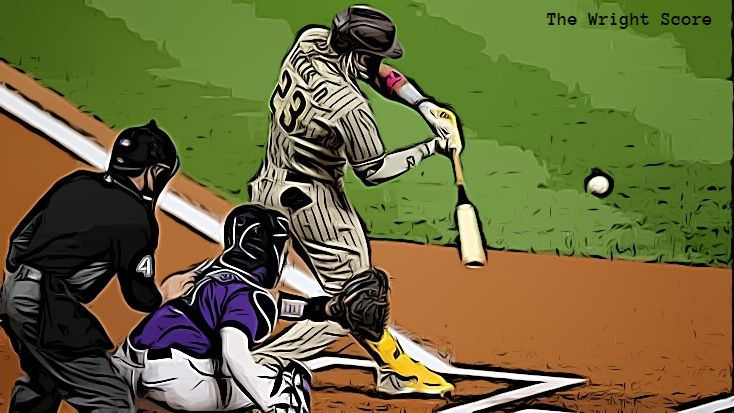
Baseball, the sport that started the whole analytical avalanche, is by far the most advanced in breaking down and predicting a game by simply looking at an excel spreadsheet. They have stats such as On-base plus slugging (OPS), which can tell you a lot about a particular hitter. It takes into account a player's on-base percentage and their slugging percentage.
For pitchers, they have Defensive Independent Pitching Statistics (DIPS). They are essential because they can consistently determine a pitcher's performance over time. They eliminate the variables that his teammate's skill level can impact. Some examples of DIPS are runs allowed, strikeouts, hit batters, walks, fly ball percentage, and ground ball percentage.
However, the most productive statistic in baseball is the RunPlusMinus™ (RPM). It is a stat that can "combine batting, fielding, pitching and runner performance into a single comprehensive value (1)." Many believers of this advanced stat claim that the winning team will always have a positive RPM total.
Football Statistics
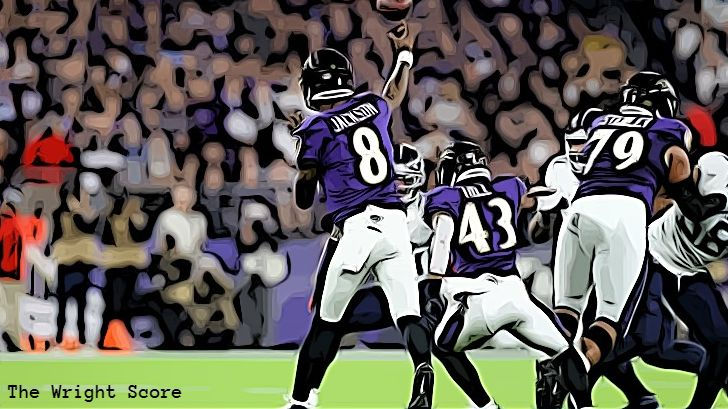
In Football, I am sure you would guess the most important stat in the game by just watching the way current teams play - Passing Yards per attempt (PYA). To be exact, it is both PYA on offense and defense. It also includes sacks in the pass attempt totals, and yards lost on a sack are subtracted from yards passing.
In fact, according to Wayne L. Winston, in his book Mathletics, if you are to run a regression to analyze a team's scoring margin with PY/A and DPY/A it will explain over 70% of the variation. It is outperforming the rest of the statistics used by over 50%. If you run a regression using running yards per attempt (RY/A) and Defensive RY/A the variation is only 6% (2).
Basketball Statistics
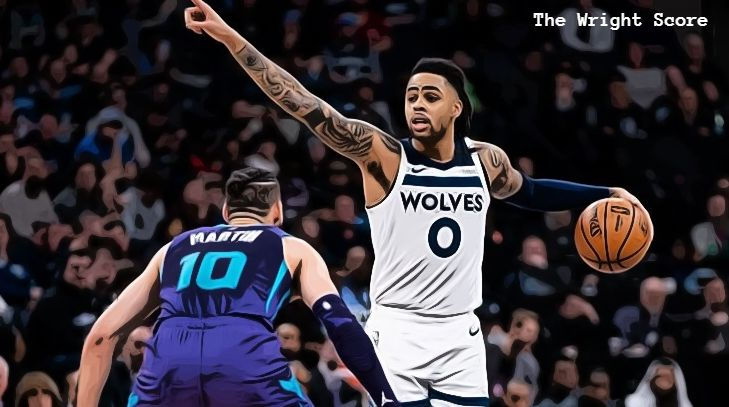
Nothing shows the influence analytics has had on the NBA more than how they are choosing to score the basketball - three-pointers. In 2012, teams averaged about 18 three-point attempts per game. This season that number is at 34, with over four teams shooting at least 40 threes a game (3).
However, the most efficient basketball stat is not three-pointer attempts or three-point percentage but the stat that explains why the three-ball craze is taking place in the first place is effective field goal percentage (eFGA). It is a statistic that fluctuates a team's field goal percentage by accounting for the fact that a three-pointer counts for three points while a normal basket is only two points. The way you measure eFGA is with the following formula: (2pt FGM + 1.5*3pt FGM) / FGA
The last nine NBA champions have all been ranked top-five or higher in eFGA.
Soccer Statistics
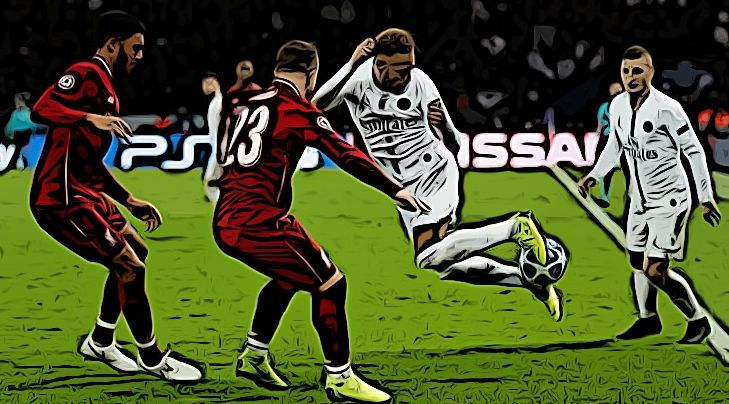
We finally made it to soccer. As mentioned in the introduction, soccer is behind when it comes to using data to impact performance and lacks in terms of what we call "advanced statistics." They rely too heavily on their basic statistics such's as goals, assist, shots, possessions, passes, corners, etc. The numbers are always fun to examine after a game. However, they do not have much significance when it comes to a team putting the ball in the back of the net.
In Figure 1, you will see the significance level of each statistic used* in the current game of soccer using a dataset from the 2019-20 EPL season. In the study, I examined the P-value of the statistics and the overall adjusted R-squared.
A p-value is determined by running a regression analysis. If a variable (stat) has a P-value score of 0.05, it is considered to be significant. The " R-Squared is a statistical measure in a regression model that determines the proportion of variance in the dependent variable that can be explained by the independent variable."(4)
The regression analysis formula used in R-studio to determine these finding is here: lm(Score~Poss+Corners+Crosses+Fouls+Inter+NumPass+NumShots+SucPass+SucShots+Touches).
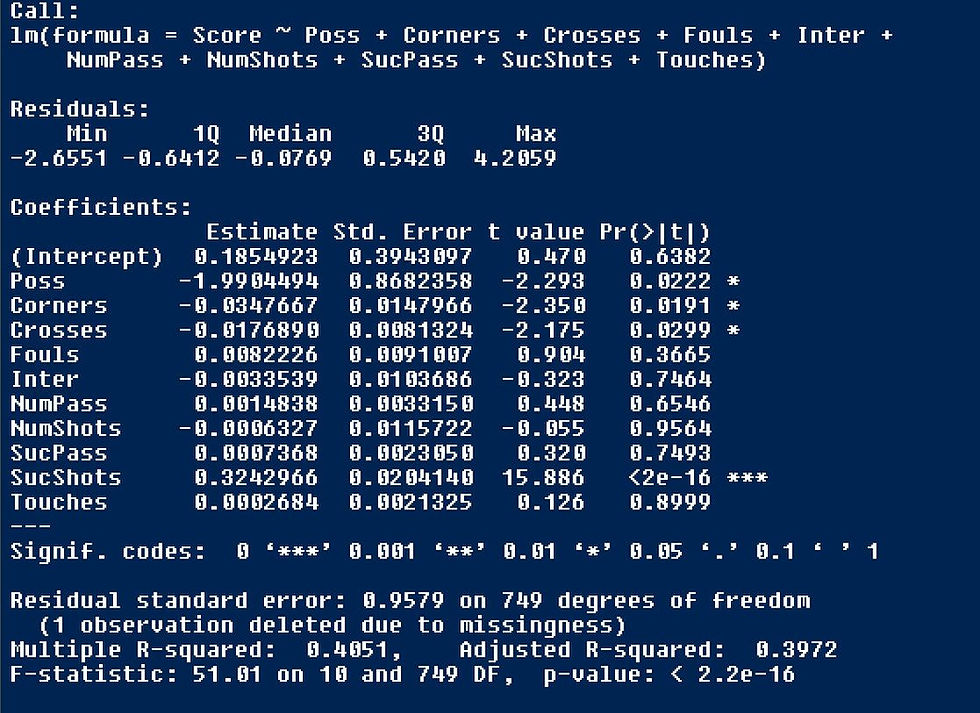
As you can see above in Figure 1, four statistics out of the eleven that were analyzed are considered to be significant in terms of having a p-value score of 0.05 (5%) or less, and 'successful shots' (SucShots) was highly significant with a P-value score of less than 0.001 (0.01%).
However, the adjusted R-squared is just 0.3972 (39.72%). This means the statistics that were examined only account for 39.72% variance when it comes to scoring a goal. Soccer will need to create new stats to raise their adjusted R-squared and find more significant variance amongst why a goal happens ----- similar to the Football section where it was shared that PY/A and DPY/A explain over 70% of the variation for a team's scoring margin.
Another reason that makes the sport of soccer so hard to analyze is none other than luck. Many times one event can determine the outcome of a 90-minute game. It is not rare for a team to dominate possession but lose1-0 because of a counterattack.
You can examine whether a team is lucky or unlucky during a season by examining their 'expected goals' xG. This newly popularized statistic is used to assess the type of shots players are taking. It looks at the history of all the shots ever taken and where they took them on the field to determine the probability that a player will score.
For example, if Neymar takes a shot from inside the six-yard box, his expected goals would be around 0.92 (92%), where if he was to take a shot from outside the 18, his expected goals could drop down to about 0.35 (35%).
The reason luck comes into play is that no xG is ever going to be 1.0 (100%) before kicking the ball. There is always the possibility of a player missing. Think back to the fluke miss Raheem Sterling had during last year's UEFA Champions League against Lyon. You would expect him to make that 99% of the time, but the 1% will always be there, and sometimes it prevails.
Below you will see a tweet from the xGPhilosophy, showing a visualization of where teams would be in the EPL standings if you based it off average xG on the year to where they are placed in the table. Brighton & Hove Albion F.C. would be fighting for a champions league bid if games were decided off xG, but instead, they are hoping to avoid relegation.
Although soccer is quite behind when it comes to using advanced analytics, many organizations are looking to change that. StatsBomb 360 recently made advancements to their data collection product. They developed technology that can track the entirety of the field, allowing them to evaluate the distances between players and determine each possession's optimal passes. This week, Liverpool became the first team to sign up for Statsbomb 360 and join the new wave of soccer analytics via skysports.com.
I predict we will see a significant change in soccer's playstyle within the next couple of years, similar to how we see the three-point revolution in basketball.
Notes:
* Statistics used in the Regression: Goal's, Possession, Corners, Crosses, Fouls, Interceptions, Number of Passes, Number of shots, success full Passes, Successful Shots, Touches.
(1) Moore, J., Ph.D. (2018, March 21). The best baseball statistic. Retrieved March 12, 2021, from https://medium.com/runplusminus/the-best-baseball-statistic-842a268b2f86
(2) NBA team three pointers Attempted per game. (2021). Retrieved March 13, 2021, from https://www.teamrankings.com/nba/stat/three-pointers-attempted-per-game
(3) Winston, W. L. (2012). Mathletics: How gamblers, managers, and sports enthusiasts use mathematics in baseball, basketball, and football. In Mathletics: How gamblers, managers, and sports enthusiasts use mathematics in baseball, basketball, and football (p. 128). Princeton, NJ: Princeton Univ. Press.
(4) R-squared - definition, interpretation, and how to calculate. (2020, June 17). Retrieved March 18, 2021, from https://corporatefinanceinstitute.com/resources/knowledge/other/r-squared/#:~:text=R-Squared%20(R%C2%B2%20or%20the%20coefficient%20of%20determination)%20is,that%20can%20be%20explained%20by%20the%20independent%20variable
Opmerkingen